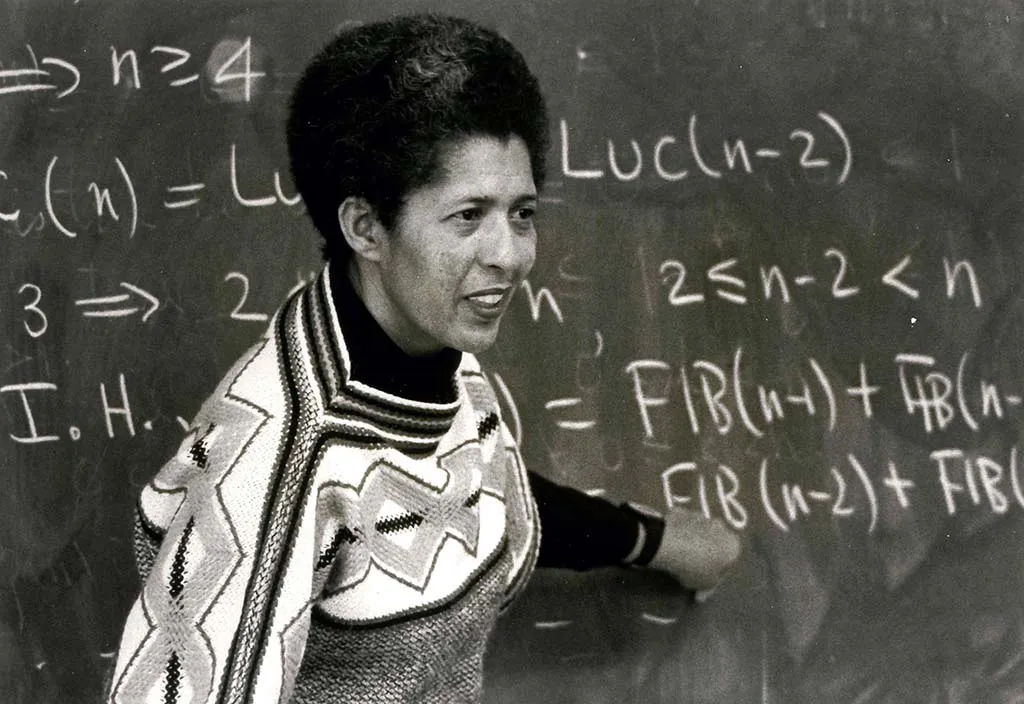
By Joy Lisi Rankin
In the 1930s Dr. Gertrude Blanch led the important Mathematical Tables Project, a nearly 450-person effort to compute logarithmic, exponential, and other calculation results essential to American government, military, finance, and science. After earning her doctorate in mathematics at Cornell, she led new approaches to computation and published volumes of tables and calculations in scientific journals. Despite her contributions, Blanch did not appear as the author of the papers she wrote. For the majority of her time on the project, her male supervisor Arnold Lowan instead received credit.
This is a lasting injustice: women, Black, Brown, and Indigenous people, people with disabilities, and other historically excluded individuals have long made crucial contributions to science, technology, and engineering, yet their labor has been continuously erased. These erasures deny contributors of their expertise, labor, community standing, and ultimately, their power. They have very real material consequences; contributions unrecognized are contributions that are not compensated. These erasures also rob us of our histories, building false narratives that justify White supremacist patriarchy as “natural” and “evidenced.”
I lead the Gender, Race, and Power in AI Program, which works to mobilize history to make the inequitable power structures on which AI technologies are built both visible and actionable. Erasure is not merely an issue of representation, but a foundation on which systemic racism, misogyny, and inequity rely. AI reinforces inequality, and that inequality has a long history. The question of whose work is seen, valued, recognized, and rewarded is not a new one, and below I outline five historically harmful forms of erasure that have helped lead us to where we are today, facing vast inequities in tech and beyond.
First, countless women like Blanch, Black people, and others who’ve borne the harms of marginalization have not historically received credit for their work. Historians and journalists alike have documented many examples where White men claimed or received publication, scientific, cultural, or public memory credit for work done by others.
Second, the work of Black people, women, and other historically excluded people has been deemed less valuable or less significant, not because of the work itself but rather because of the identities of the people performing it. Despite the fact that the Math Tables Project produced outstandingly accurate and complex tables, and ultimately contributed to the American effort during World War II, many (male) scientists, engineers, and mathematicians looked down on it at the time, some because they considered it a New Deal “boondoggle,” and others because it employed not just women but also people with disabilities. Until recently, the Black women upon whom the American space program — and its astronauts — depended had been written out of history. Likewise, the history of Black employment — and racism — at IBM and the contributions of Black computing pioneers had long since been forgotten. Although Navajo women played a key role in manufacturing semiconductors for industry giant Fairchild, their labor was devalued by being constituted as a feminine “labor of love.” Latina/o information workers have been essential to the telecommunications industry since the 1970s, yet their contributions have been neglected, even as they’ve been heavily surveilled by their employers. This history is still with us: in the past decade, the differentiation between front-end, back-end, and full stack developers arose as a way to diminish the work of women who were coding.
Valuing, esteeming, and compensating labor based on who is doing it rather than the work itself is (unfortunately) nothing new, and has shaped who gets access to acclaim and resources, and who doesn’t. During the 19th and early 20th centuries, most public school teachers in the United States were men, and the profession was well-paid and viewed with respect. As more women became teachers over the course of the twentieth century, their work was devalued. Today, teachers are paid only about 70% of what comparably educated college graduates earn in the United States; many teachers work second jobs to survive.
Third, as computing and related STEM fields have become more prestigious, women, BIPOC, and others who’ve faced discrimination have been actively pushed out. While women formed the heart of the nascent British technology industry during World War II and the postwar years, the British Civil Service decided that men would be better suited for those jobs. Rather than promote qualified and highly skilled women, Britain pushed out the women and brought in unqualified men. The United States suffered similar losses: In the mid-1980s, nearly 40% of undergraduates earning computer science degrees were women, yet by 2010 that number had plummeted to 15%. Some of the forces that pushed those who weren’t White men out of American computing include returning WWII veterans who displaced women from science and engineering jobs.
Fourth, the culture of the elite technology industry (whether IBM in the 60s, Microsoft in the 90s, or Google today) has continually favored masculine traits among its employees. So-called aptitude tests for programming did nothing more than embody a narrow view of programming as “straightforward, mechanical, and easily isolated” (p64). During the 1960s, tech companies increasingly used personality profiles to identify programmers, and although programmers generally resembled other white-collar professionals, their seeming “disinterest in people” (p69) engendered a new, masculine programmer persona. Those personality profiles merely reflected the personality traits of those already employed. More recently, some have criticized this particularly Silicon Valley notion of meritocracy as, instead, mirror-tocracy. Elite employers use video games to evaluate candidates, which (among other things) pre-selects for candidates who enjoy and are comfortable with gaming. Indeed, the mirror-tocracy contributed to Amazon’s creation of a hiring algorithm biased against women, which was built based on histories of Amazon’s hiring practices.
Finally, even when they perform vital technological work, women, Black people, and others who fall outside of the White man programmer stereotype are presented not as crucial laborers and producers but rather as domestic or inept consumers and users. When John Kemeny, who later became President of Dartmouth College, spoke at the dedication of the College’s new computing center in 1966, he invoked women in computing by describing housewives using in-home computing terminals to program their chores, prepare balanced menus for their family, or place shopping orders. But while Kemeny was talking about housewives computing balanced meals, women were already working in key roles on the Dartmouth network. Janet Price, Diane Hills, and Dianne Mather were all application programmers, and Nancy Broadhead managed user services, to name just a few. These were not exceptions to the norm, but rather represented the range of possibilities for women doing professional computing in the 1960s.
The data we do have on the current state of diversity in the field indicate minimal progress. Moreover, there is reason to question the figures that companies themselves provide. For example, Google instituted what it calls the “plus system,” double-counting multiracial employees as belonging to multiple racial categories. This change in methodology inflates the company’s progress toward its diversity hiring goals, and makes it harder to compare current diversity data to past data. It also highlights the fact that much of what we know about diversity is what these companies choose to report.
Most mainstream diversity in tech “solutions” focus on pipelines or pathways, which assume that the problems causing inequality in tech lie on the path to the job, not with the company itself. This actively ignores the fact that there have always been Black people, women and others who’ve faced discrimination doing this work. We need structural change that addresses the systems, cultures and norms, policies and practices, attitudes of existing workers, and the other myriad ways that the tech industry continues to marginalize Black people, women, disabled people, and others who already suffer the harms of historic inequity. As one expert notes, “Systemic change is difficult, and it is unsettling to those who have existed in the established system. It is not surprising that…technology companies were more engaged in recruiting new women and minority hires rather than changing the practices and environments within their organizations so as to improve retention and advancement of women and underrepresented minorities” (p11). Many who have benefitted from inequity are firmly invested in its preservation. Righting inequity means that some in power will lose it.
The Gender, Race, and Power in AI Program looks to past and current social, political, and economic justice movements for paths forward. We seek to emulate “group-centered leaders” including Ella Baker and Septima Clark. These brilliant and heroic Black women were instrumental in the grassroots activism that propelled America’s long Civil Rights Movement. They emphasized community education and increasing literacy as foundations for social change. In turn, the Gender, Race, and Power program aims to increase literacy about power imbalances in AI. Baker and Clark’s fight against anti-Black racism resonates today, as young Black activists lead the nation’s uprising against White supremacist violence following the police killings of George Floyd, Breonna Taylor, and so many other Black people.
AI systems draw on our past and our present to create models of the world that are shaping lives and opportunities. These models encode all of the assumptions, inequities, and oppressions of our social and political systems, then and now. There is no escaping this. This means that the AI field in particular must address and account for history. It must expand to include disciplines capable of accurately teaching this history, and recognize that this history moves through the present. Responsible AI must be as much backward-looking and past-reckoning as it is forward-thinking and future-planning.