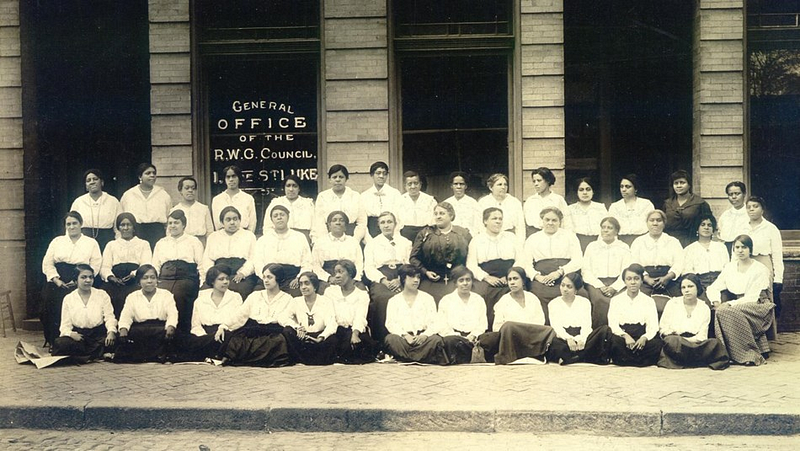
By Sarah Myers West, Postdoctoral Researcher, AI Now Institute
Image: Credit discrimination in the US has a long history. Maggie Lena Walker was early to identify — and resist — biased lending practices. Photo credit: Courtesy of National Park Service
Last week, software engineer David Heinemeier Hansson took to Twitter when Apple Card approved his application and gave him a credit limit 20 times higher than his wife, Jamie Heinemeier Hansson. Janet Hill, who is married to Apple co-founder Steve Wozniak, received a credit limit 10% of her husband’s — not even enough to buy a plane ticket.
The culprit, according to David? A sexist black box algorithm.
The pair’s accounts detailing their experiences with Apple Card set off an outcry over algorithmic discrimination online, prompting presidential candidate and founder of the Consumer Financial Protection Bureau Elizabeth Warren to call for Apple and Goldman Sachs, the bank behind the card, to pull it down if they couldn’t fix the problem. The Senate Committee on Finance and New York State Department of Financial Services both announced they will launch investigations.
If only all claims of algorithmic bias were taken so seriously. AI-driven discrimination impacts us all, whether or not we use Apple Cards. We see it in healthcare, when UnitedHealth Group used a racially biased algorithm that steered black patients away from getting the quality healthcare they needed. This system was in active use for years before researchers identified the discrepancy. We see it in hiring practices. An advocacy group recently issued a complaint to the Federal Trade Commission over a company that scans the faces and voices of job applicants, calling on the agency to investigate whether it is engaged in ‘unfair and deceptive business practices’.
For at least a decade, researchers, journalists, activists, and even tech workers have been sounding the alarm about biased AI systems. Many of those pioneering this work are people of color, and they predominantly identify as women or non-binary. They have engaged in detailed work to detect and prove bias in advertising networks, search engines, facial recognition, welfare systems, and even algorithms used in criminal sentencing.
They’re also most likely who Google Chairman Eric Schmidt had in mind when he appeared before an audience at Stanford and said, “We know about data bias. You can stop yelling about it.”
Schmidt’s offhand statement underlined an ugly truth — tech companies know they have a problem on their hands, and that algorithmic discrimination is deeply embedded in the systems they are unleashing into the world. It’s enough of an issue that Microsoft listed reputational harm due to biased AI systems among the company’s risks in its latest report to shareholders. But the industry thus far seems unwilling to prioritize solving these issues over their bottom line.
Addressing this won’t be easy, as Goldman Sachs is finding. But just because things are hard doesn’t mean they’re not worth doing. The company responded to the allegations of bias, stating that credit decisions were not based on gender, race, age, and sexual orientation, or any other basis prohibited by law. We only have their word to go on, which is part of the problem. But what they’ll likely find — if they haven’t already — is that removing these factors from the decision-making process may be harder than it sounds. Patterns of inequality on the basis of gender and race may still influence the outcome of a credit application, even when the applicant doesn’t provide this information outright.
As Kate Crawford, co-founder of the AI Now Institute, put it, “Bias is more a feature than a bug of how AI works.” Introducing AI into the credit assessment process doesn’t make it fairer or more objective — it simply adds layers of complexity to a system that already has a long history of discrimination.
One place the industry can start is by looking closer to home. The AI industry is notably non diverse, even less so than the tech industry at large. One study found that only 18% of authors in leading AI conferences were women, while another showed that 80% of AI professors are men. We lack comprehensive data on representation by race, ethnicity, or ability, but indicators suggest things look much worse. Without this diversity of experience, it will be difficult for those developing these systems to identify and mitigate the harms they produce.
But often ‘diversity’ in tech is treated as merely a marketing exercise, centered around “Women in Tech” initiatives that privilege white women above others. The challenges go far beyond, from barriers to entry to toxic experiences within AI spaces. As Jamie Heinemeier Hansson put it, “This is not merely a story about sexism and credit algorithm black boxes, but about how rich people nearly always get their way. Justice for another rich white woman is not justice at all”. This is not just about credit algorithms: it is past time for the tech industry to listen to those who are showing companies where they are going astray by perpetuating discrimination in their products and workplace environments.