Tamara Kneese, Data & Society Research Institute
Download full report here.
Introduction
In the second half of 2023, generative AI is dominating headlines. Policymakers, technologists, and activists are all grappling with its potential implications for communities and the planet. Integrating LLMs (large language models) into search engines may multiply the carbon emissions associated with each search by as much as five times. At a moment when climate change is already having catastrophic effects and the final 2023 IPCC report indicates that the current gap between net zero pledges and global GHG (greenhouse gas) emissions reductions means that it is highly likely that the earth’s warming will exceed 1.5 degrees Celsius by the end of the century, the current hype around AI seems especially dangerous. Simultaneously, generative AI poses risks to workers in a number of fields, continuing a long trend of labor exploitation through apparent automation. Despite the perception that generative AI will automate jobs and render knowledge workers obsolete, research shows time and time again that humans, and globally dispersed, poorly paid annotators, are still operating behind AI. A difficult to parse supply chain of ecological extraction and labor exploitation facilitates the apparent magic of AI.
In this report, I examine the intersection of these two issues in AI: climate and labor. Part I focuses on the relationship between AI labor supply chains and internal corporate workplace practices and hierarchies. How are researchers and developers grappling with the complex problem of calculating carbon footprints in machine learning while assessing potential risks and impacts to marginalized communities? In an industry dominated by OKRs (objectives and key results) and quantifiable success metrics, the importance of carbon accounting or other data collection and analysis tends to take precedence over other forms of action. In other words, even with the introduction of regulations demanding that companies measure and report their carbon emissions, it is unclear if measurement alone is enough to actually reduce carbon emissions or other environmental and social impacts. In Part II, I examine organizing campaigns and coalitions, in historical and contemporary contexts both inside and outside of the tech industry, that seek to connect labor rights to environmental justice concerns. Part I takes stock of the problem and Part II offers some potential steps toward solutions.
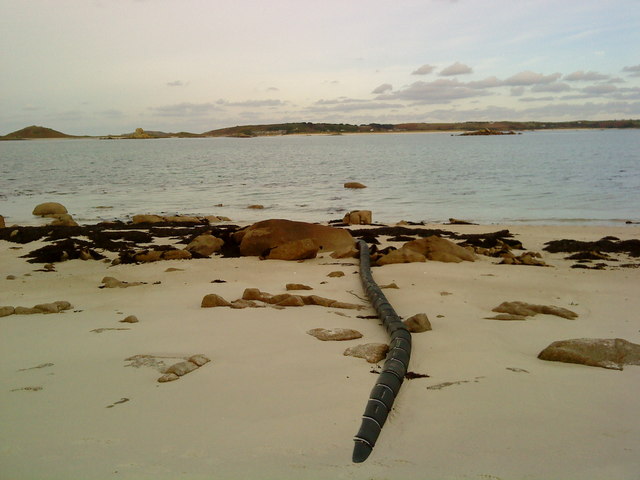
Assessing Impact: Carbon and Beyond
New waves of legislation are scrambling to address the various potential effects of powerful LLMs. Stanford researchers assessed foundation models according to a range of criteria set forth by the European Union’s AI Act draft, including energy, compute, risks and mitigations, and data governance. In their Artificial Intelligence Risk Management framework, published in January 2023, and in their newly launched Trustworthy and Responsible AI Reference Center, the National Institute of Standards and Technology references the environmental impact of AI: “AI technologies, however, also pose risks that can negatively impact individuals, groups, organizations, communities, society, the environment, and the planet.” Despite these developments, more robust connections between environmental and social harms, and labor rights in particular, are sorely needed.
Researchers within technology companies and in academia are also finding new ways of calculating the environmental toll of AI. Some technologists call for the development of a greener machine learning focused on energy efficiency, benchmarking tools, and carbon reporting. In a co-authored paper, Alexandra Sasha Luccioni of the AI startup Hugging Face has advocated for life cycle analysis, considering embodied emissions tied to the manufacturing of the equipment needed to produce and train AI in addition to the machine learning training itself, adding the emissions tied to deployment and use to those involved with disposal. Researchers at the University of California Riverside and the University of Texas Arlington have written a paper examining the water footprint of AI. In an interview, lead researcher Shaolei Ren emphasizes that carbon efficient and water efficient times of day might be at odds, so developers must consider the effects of scheduling their model training for specific times of the day or in particular locations and carefully weigh their options. It is crucial to consider carbon emissions in tandem with other environmental factors, including water use, and social repercussions.
In their famous “Stochastic Parrots” paper, researchers Emily Bender, Timnit Gebru, Angelina McMillan-Major, and Margaret Mitchell examine the environmental and ethical issues associated with ever-larger language models. Most cloud compute energy sources are not from renewable energy sources, and even renewable energy has an environmental toll because of the rest of the supply chain and the life cycle of AI development. Bender et al. explicitly connect the environmental impact of machine learning to some of its other ethical implications, including its contribution to perpetuating inequalities, inadvertently harming LGBTQ and Black people based on filtering mechanisms, and advancing harmful ideologies. Large datasets do not guarantee diversity. People also attribute communicative intent to LLMs, even though they are not humans and do not have consciousness. LLMs tend to benefit those who already have the most power and privilege. As they argue, “It is past time for researchers to prioritize energy efficiency and cost to reduce negative environmental impact and inequitable access to resources — both of which disproportionately affect people who are already in marginalized positions.”
It is noteworthy that Mitchell, the founder of the AI ethics team at Google, and Gebru, a renowned Black woman researcher on the same AI ethics team, were fired from Google because of the company’s discomfort with the paper’s arguments. While companies espouse values about DEI (diversity, equity, and inclusion), ethics, and sustainability, workers with these expertise and sometimes entire dedicated teams are often some of the first to be impacted by layoffs. As Sanna Ali et al. argue in their 2023 FAccT paper, if there is a lack of institutional support for AI ethics initiatives, then the burden falls on individuals to advocate for their work. At every step, ethics workers are inhibited by a company’s focus on product innovation, quantitative metrics, and perpetual reorgs that disrupt workflows and relationships.
In tech circles and climate pledges, there is a tendency to focus on calculating, measuring, and reporting GHG emissions at the expense of other factors. There is a problem with fetishizing carbon, particularly when carbon offsets are another point of financialization and exploitation, such as when carbon offsets are used to justify colonialism in the Amazon in the name of regenerative finance. STS scholars Anne Pasek, Hunter Vaughan, and Nicole Starosielski thus call for a more relational approach to thinking about the carbon footprint of the ICT industry: “Rather than seeking to evaluate sectoral performance as a whole, and thus overcome vast data frictions in assessments at a global scale, relational footprinting identifies specific differences between discrete and measurable local elements and suggests how these differences might be leveraged for climate mitigation.” They advocate for a “reorganization of global infrastructures in order to leverage regional energy differences.” Too often, for tech companies, searching for the perfect data, or the act of measurement itself, is the end of the story.
There are many differing ways of measuring the carbon impact of various technologies, including AI. But that doesn’t necessarily take into account global differences and, even moreso, the downstream effects on marginalized communities. Some researchers, including Bogdana Rakova, Megan Ma, and Renee Shelby, are attempting to reconceptualize AI as ecologies, imagining a more transparent form of algorithmic accountability based on feminist principles and solidarity. Rakova calls for a feminist disruption of AI production, focusing on the power of speculative frictions: rather than thinking of humans as sources of friction in automated systems that should be made invisible or rendered obsolete, Rakova urges AI practitioners to take up a more cautious form of technological production, drawing on a Mozilla Festival workshop that included diverse voices in thinking about how AI might intervene in environmental justice problems and what it would mean for humans and ecosystems to intentionally slow down AI production through friction.
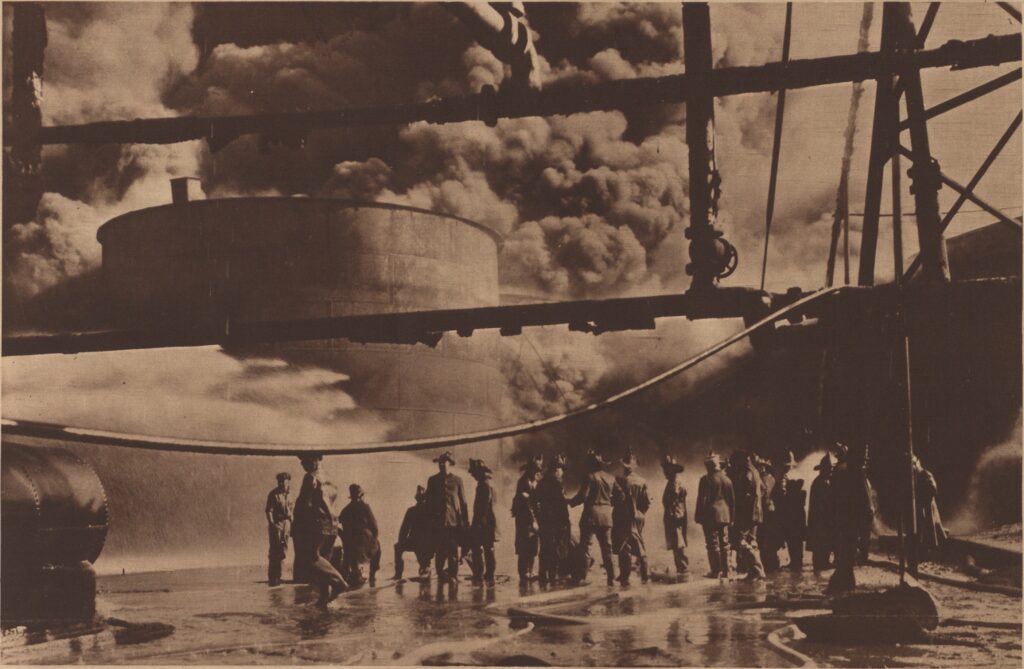
Workflows and Hierarchies in AI Production
Aside from global power asymmetries, there are clear power differentials between corporate leaders and rank-and-file developers within major tech companies, and this creates a disconnect between corporate sustainability initiatives and workplace climate activism.
Corporate net zero goals are built on speculative, and often empty, promises. Most companies are failing to meet their targets and would have to redouble their emissions reductions efforts to be carbon negative by 2030. At COP27, U.N experts released a report proposing new standards to counteract corporate greenwashing. Carbon offsets are essentially scams, another example of a technologically-driven solution to a social problem. In some cases, carbon offsets actively harm marginalized people and endangered species in parts of the world that are already facing catastrophic climate impacts.
Some technologists hope to use innovation to decarbonize the industry, as corporate responsibility staff, IT managers, and engineers attempt to measure, report, and reduce carbon emissions across the global supply chain. When it comes to high-energy workloads like machine learning, making emissions legible through telemetry is especially crucial. Developers and managers cannot make informed decisions without data. Carbon aware software helps AI developers understand the relationship between their workflows and the energy grid, letting them know if there is an optimal time of day to train their models depending on where they are geographically situated. But, aside from the developer’s relationship to time and place, power differentials also influence their decisions, as managers and C-suite members might have other priorities, including cost, performance, and shipping a product as quickly as possible.
Technologists have found ways of mitigating the carbon cost of machine learning training. Carbon intensity is a major factor. Part of why Hugging Face’s BLOOM model has a smaller carbon footprint than GPT-3 and other models is the lower carbon intensity of the energy source used for training, which means selecting energy source locations according to the availability of renewables and choosing to train models at optimal times of day. Batch scheduling involves getting developers to choose times of day and locations that are optimal according to when the grid has more renewables available. But developers don’t always have control over these conditions. They are often pressed for time and face other workplace pressures. They might have managers who do not prioritize these assessments. At the same time, examining these decision-making processes in corporate labs provides insight into relationships between IT infrastructure, power differentials and workplace hierarchies, and internal workflows. Carbon awareness is in some ways an entry point to STS-informed, ecological approaches that go beyond footprinting or meeting net zero goals.
At most companies, management’s focus is on product development and prototyping, not on downstream effects. It’s hard enough to get C-level buy-in for thinking about the developer, the most obvious human-in-the-loop from their perspective, let alone the generic end user, and forget about anyone or anything outside of that narrow definition of human-computer interaction.
There are issues with only viewing one small part of the AI life cycle. What about the mining and other forms of labor that go into manufacturing and production, or the winding down of AI systems and associated e-waste? The true impact of AI production and use is connected to the larger supply chains, and the poor working conditions, of the entire ICT industry: from the cobalt miners in the Democratic Republic of Congo and Foxconn manufacturing workers in China to e-waste labor and caste politics in India. Ada Lovelace Institute has put out a primer on AI supply chains, including policy recommendations for addressing the thorny problems of accountability in foundation model supply chains and gaps between open source and proprietary models.
Such complications also impact internal workplace practices. As David Gray Widder and Dawn Nafus observe in their paper about AI ethics and the supply chain, a convoluted global supply chain can cloud developers’ sense of agency, and thus their sense of responsibility. Ethics checklists aren’t necessarily effective when software practitioners feel that they have little control over certain elements of their work: “Obligations and dependencies also look different depending on whether one is looking upstream or down, and it is crucial to recognize these social locations when creating deeper expectations of responsibility. We show below that this social reality has created conditions where interventions fall through the cracks between actors, and has defined other chains of relations (business, personal reputations, user experience, etc.) as secondary or out of scope.” And when company leadership espouses AI ethics as a core belief, some developers question if this is a form of ethics washing, wondering if their actions indeed back up their claims. Widder and Nafus also point to hierarchies within AI labor, as developers distinguished between paperwork and more prestigious forms of work.
Conclusions: AI and labor/climate ecologies
As AI is integrated into more and more existing infrastructures, the question becomes: do we really need AI for that? What are companies building and why? Who is it for? Beyond the end user, who are the other networks of people, environments, ecosystems, that are going to be affected up and down the supply chain and through its associated labor practices?
Some might question the wisdom of producing energy-intensive chatbots at a time when climate action is desperately needed: generative AI seems especially pernicious because aside from its carbon cost and over environmental and labor impacts, it is a distraction from the very real existential threat of climate change. Concerns over the existential threat of AI also eclipse attention that should go to climate matters. Geoffrey Hinton, a supposed ‘godfather of AI’ who recently resigned his position at Google, has stated that AI “might end up being more urgent” than the risk of climate change and that climate change is an easier problem to solve. Such arguments can be used to put more financial resources, and tech press and regulatory attention, towards AI rather than taking necessary steps in mitigating climate change.
There is a general problem with the disconnect between corporate responsibility, and discourses about circularity or sustainability, and actual corporate models of production and the realities of endless growth. Just a handful of multinational companies have all of the power. They own and run the cloud. Power structures in corporate AI development determine who has access to the compute needed to develop, train, and deploy these technologies.
Too often, greenness is bracketed off from other justice concerns and from historically derived, colonialist power structures. Carbon accounting and AI monitoring in various climate contexts or the analysis of open source climate-related data doesn’t translate into action unless there is power behind it. Does AI transparency help if there is no one empowered to change workflows and systems?
There is not a singular technical solution to a social problem. AI will not solve climate change. Tooling in general won’t solve it, either. So what can rank-and-file workers within major companies do to push for more holistic, social justice-oriented interpretations of sustainability in their workplaces and communities? Part II will attempt to answer this question.