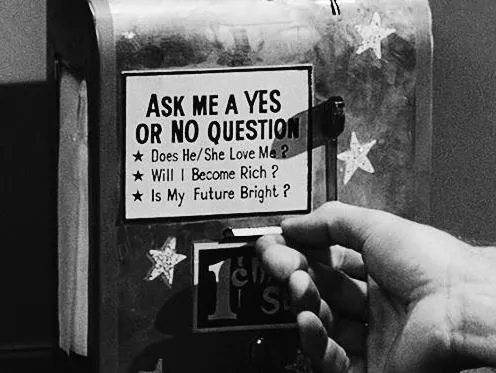
Algorithmic Impact Assessments: Toward Accountable Automation in Public Agencies
Feb 21, 2018
Update 4/9/2018: We have released a new report describing our proposal for Algorithmic Impact Assessments in full detail. The report describes how affected communities and stakeholders can use our framework to assess the use of AI and algorithmic decision-making in public agencies and determine where — or if — their use is acceptable.
In the coming months, NYC Mayor Bill de Blasio will announce a new task force on “Automated Decision Systems” — the first of its kind in the United States. The task force will recommend how each city agency should be accountable for using algorithms and other advanced computing techniques to make important decisions. As a first step toward this goal, we urge the task force to consider a framework structured around Algorithmic Impact Assessments (AIAs).
Automated decision systems are here, and are already being integrated across many core social institutions, reshaping how our criminal justice system works via risk assessment algorithms and predictive policing systems, optimizing energy use in critical infrastructure through AI-driven resource allocation, and changing our educational system through new teacher evaluation tools and student-school matching algorithms. And these are merely what journalists, researchers, and the public record expose — to date, no city in the US has explicitly mandated that its agencies disclose anything about the automated decision systems they have in place or are planning to use.
While these systems are already influencing important decisions, there is still no clear framework in the US to ensure that they are monitored and held accountable.¹ Indeed, even many simple systems operate as “black boxes,” as they are outside the scope of meaningful scrutiny and accountability. This is worrying. If governments continue on this path, they and the public they serve will increasingly lose touch with how decisions have been made, thus rendering them unable to know or respond to bias, errors, or other problems. The urgency of this concern is why AI Now has called for an end to the use of black box systems in core public agencies. Black boxes must not prevent agencies from fulfilling their responsibility to protect basic democratic values, such as fairness and due process, and to guard against threats like illegal discrimination or deprivation of rights.
With this in mind, and drawing on several ongoing research efforts, AI Now is proposing an early-stage framework centered on Algorithmic Impact Assessments (AIAs). This broad approach complements similar domain-specific proposals, like Andrew Selbst’s recent work on Algorithmic Impact Statements in the context of predictive policing systems. These frameworks in turn draw on the history and development of assessments in other areas — such as environmental policy, privacy law, and data protection in the EU — and build on growing and important research that scientific and policy experts have been developing on the topic of algorithmic accountability.² AIAs begin to shed light on these systems, helping us to better understand their use and to determine where they are and aren’t appropriate, both before they are deployed and on a recurring basis when they are actively in use.
AIAs strive to achieve four initial goals:
- Respect the public’s right to know which systems impact their lives and how they do so by publicly listing and describing algorithmic systems used to make significant decisions affecting identifiable individuals or groups, including their purpose, reach, and potential public impact;
- Ensure greater accountability of algorithmic systems by providing a meaningful and ongoing opportunity for external researchers to review, audit, and assess these systems using methods that allow them to identify and detect problems;
- Increase public agencies’ internal expertise and capacity to evaluate the systems they procure, so that they can anticipate issues that might raise concerns, such as disparate impacts or due process violations; and
- Ensure that the public has a meaningful opportunity to respond to and, if necessary, dispute an agency’s approach to algorithmic accountability. Instilling public trust in government agencies is crucial — if the AIA doesn’t adequately address public concerns, then the agency must be challenged to do better.
Goal One: Provide the public with information about the systems that decide their fate
A fundamental aspect of government accountability and due process is notice of how our rights are being affected, and by which government agencies and actors. When automated systems play a significant role in government decisions, they should be disclosed.
Thus, as a first step, Algorithmic Impact Assessments would require each agency to publicly list and describe all existing and proposed automated decision systems, including their purpose, reach, and potential impacts on identifiable groups or individuals. This requirement by itself would go a long way towards shedding light on which technologies are being deployed to serve the public, and where accountability research should be focused. Similar provisions are already part of laws in the U.S. such as the Privacy Act of 1974, and have been proposed in emerging local ordinances such as one in Santa Clara County and another in Oakland that are focused on privacy.
Of course, in order to make disclosure meaningful, “automated decision making” must be defined in ways that are both practical and appropriate. An overly-broad definition could burden agencies with disclosing systems that are not the main sources of concern. If a public servant uses a word processor to type up her notes from a meeting where some key decisions were made and then checks them with the program’s “automated” spell-checker, her agency should not have to perform an AIA for that spell-checker. On the flipside, an overly-narrow definition could undermine efforts to include high-profile systems like those deciding which students are admitted to specialized high schools or how housing opportunities are allocated.
It is also essential that “systems” are defined in terms that are broader than just their software — AIAs should cover human factors too, along with any input and training data. Bias in automated decision systems can arise as much from the human choices on how to design or train the system as they can from human errors in judgment when interpreting or acting on the outputs.³ Evaluating a predictive policing system, for instance, is not just a matter of understanding the math behind its algorithm; we must also understand how officers, dispatchers, and other decision-makers take its outputs and implement them in both policy and everyday practices.
To ensure that we draw an appropriate boundary around automated decision systems, Algorithmic Impact Assessments must set forth a reasonable and practical definition of automated decision making. This process of defining and specifying such systems would help build agency capacity for the procurement and assessment of future systems, as experience with AIAs would help guide Requests for Proposals, budgeting, and other key milestones.
Crucially, agencies would not be working alone to create these definitions. In order for AIAs to be effective, agencies must publish their definition as part of a public notice and comment process whereby individuals, communities, researchers, and policymakers could respond, and if necessary challenge, the definition’s scope. This would allow push back when agencies omit essential systems that raise public concerns.
Consider the example of an education agency. A reasonable agency’s definition should include an automated decision system such as the Educational Value-Added Assessment System, used by many jurisdictions for automated teacher evaluations. We might expect that the text of that agency’s definition would include something like the “systems, tools, or statistical models used to measure or evaluate an individual teacher’s performance or effectiveness in the classroom.” In a criminal justice agency, similar wording might yield a definition that includes “systems, tools, or statistical models used to measure or evaluate an individual criminal defendant’s risk of reoffending.”
A definition that focuses on individual profiling has a precedent. In the EU’s General Data Protection Regulation (GDPR), automated profiling is defined as “any form of automated processing of personal data consisting of the use of personal data to evaluate certain personal aspects relating to a natural person, in particular to analyse or predict aspects concerning that natural person’s performance at work, economic situation, health, personal preferences, interests, reliability, behaviour, location or movements.”⁴
The GDPR language may be a good starting point but will require some shaping to match the appropriate contexts. And in other contexts it may not be sufficient. Some predictive policing tools, for example, don’t necessarily constitute “profiling individuals,” and instead focus on locations, using statistics to try to understand and predict crime trends, with the potential for disparate impact. A definition might then have to account for “any systems, tools, or algorithms that attempt to predict crime trends and recommend the allocation of policing resources” in non-individualized terms. In general, any definition should be sure to cover systems that might have a disparate impact on vulnerable communities, and to pay careful attention to how broad terms, like “automated processing,” are specified in practice.
Goal Two: Give external researchers meaningful access to review and audit systems
After internal agency processes work to publicly disclose existing or proposed systems, Algorithmic Impact Assessments should provide a comprehensive plan for giving external researchers meaningful access to examine specific systems and gain a fuller account of their workings. While certain individuals and communities may wish to examine the systems themselves, it would be unreasonable to expect that everyone has the time, knowledge, and resources for such testing and auditing.⁵ Automated decision systems can be incredibly complex, and issues like bias and systematic errors may not be easily determined through the review of systems on an individual case-by-case basis. A plan to grant meaningful access would allow individuals and communities to call upon the trusted external experts best suited to examine and monitor a system, and to assess whether there are issues that might harm the public interest.
To do this well, it’s important to recognize that the appropriate type and level of access may vary from agency to agency, from system to system, and from community to community. The risks and harms at issue in different systems may demand different types of research across different disciplines.⁷ While the right to an explanation concerning a specific automated decision could prove useful in some situations, as it is suggested in the GDPR framework, many systems may require a group-level or community-wide analysis.⁶ For example, an explanation for a single “stop and frisk” incident would not reveal the greater discriminatory pattern that the policy created in NYC, where over 80% of those stopped were black or Latino men.
Other systems may only require analysis based on inputs and outputs without needing access to the underlying source code. We believe that the best way for agencies to develop appropriate research access programs initially would be to work with affected communities and interdisciplinary researchers through the notice and comment process. Importantly, given changing technologies, the developing research field around accountability, and the shifting social and political contexts within which systems are deployed, access to a system will almost certainly need to be ongoing, and take the form of monitoring over time.
Ongoing monitoring and research access would also allow agencies and researchers to work together to develop their approaches to testing. The research around algorithmic accountability is young. We do not yet know what future tools and techniques might best keep systems accountable. External researchers from a wide variety of disciplines will need the flexibility to adapt to new methods of accountability as new technologies drive new forms of automating decisions.
To effectuate external research access, public agencies will also need to commit to accountability in both their internal technology development plans as well as vendor and procurement relationships. For example, meaningful access to automated decision systems will not be practical or feasible if essential information about the system can be shielded from review by blanket claims of trade secrecy. Agency AIAs commit each agency to ensuring meaningful review of these systems; therefore, agencies may need to require potential vendors to waive restrictions on information necessary for external review. For example, at minimum vendors should be contractually required by agencies to waive any proprietary or trade secrecy interest in information related to accountability, such as those surrounding testing, validation, and/or verification of system performance and disparate impact.
Of course, there is also a real danger that relying on external auditing will become an unfunded mandate on researchers to check automated decision systems. However, there are models that legislation could adopt to address this. An AIA framework could fund an independent, government-wide oversight body, like an inspector general’s office, to support the research and access. Or funding could be set aside for the compensation of external auditors. Fortunately, there are many options that jurisdictions could consider for their own needs. And a growing community of computer scientists, journalists, and social scientists have already proven there is an appetite for research into public automated systems.⁸
Goal Three: Increase public agencies’ capacity and expertise to assess fairness, due process, and disparate impact in automated decision systems
Access for external researchers is a crucial component of algorithmic accountability, but in parallel we need to increase the internal capacity of public agencies to better understand and explicate potential impacts before systems are implemented. Agencies must be experts on their own automated decision systems if they are to ensure the public trust. That’s why agencies’ Algorithmic Impact Assessments must include an evaluation of how a system might impact the public, and show how they plan to address any issues, should they arise.
This is an opportunity for agencies to develop expertise when commissioning and purchasing automated decision systems, and for vendors to foster the public trust in their systems. Agencies will be better able to assess the risks and benefits associated with different types of systems, and work with vendors to conduct and share relevant testing and research on their automated decision system, including but not limited to testing for any potential biases that could adversely impact an individual or group interest and any other validation or verification testing conducted. As noted above, if some vendors raise trade secrecy or confidentiality concerns, those can be addressed in the AIA, but responsibility for accountability ultimately falls upon the public agency.
AIAs would also benefit vendors that prioritize fairness, accountability, and transparency in their offerings. Companies that are best equipped to help agencies and researchers study their systems would have a competitive advantage over others. Cooperation would also help improve public trust, especially at a time when skepticism in the societal benefits of tech companies is on the rise. These new incentives encourage a race to the top of the accountability spectrum among vendors.
Increasing agency expertise through AIAs will also help promote transparency and accountability in public records requests. Today, when agencies receive open records requests for information about algorithmic systems, there is often a mismatch between how the outside requestor thinks agencies use and classify these technologies and the reality. As a result, requests may often take a scattershot approach, cramming overly broad technical terms into numerous requests in the hopes that one or more hit the mark. This can make it difficult for records officers responding in good faith to understand the request let alone provide the answers the public needs.
Even open records experts who are willing to reasonably narrow their requests may be unable to do so because of the lack of any “roadmap” showing which systems a given agency is planning, procuring, or deploying. For example, in a project out of the University of Maryland, faculty and students working in a media law class filed numerous general public records requests for information regarding criminal risk assessment usage in all fifty states. The responses they received varied significantly, making it difficult to aggregate data and compare usage across jurisdictions. It also revealed a lack of general knowledge about the systems among the agencies, leading to situations where the students had to explain what ‘criminal justice algorithms’ were to the public servants in charge of providing the records on their use.
Accountability processes such as the AIA would help this mismatch on both sides of the equation. Researchers, journalists, and concerned members of the public could use the Algorithmic Impact Assessments to reasonably target their requests to systems that were enumerated and described, saving public records staff significant time and resources. Agency staff would also gain a better handle on their own systems and records, and could then help requestors understand which documents and public records are potentially available. This alignment would increase efficiency, lower the agency burden of processing requests, and increase public confidence.
Agencies could also use the AIA as an opportunity to lay out any other procedures that will help secure public trust in such systems. If appropriate, the agency might want to identify how individuals can appeal decisions involving automated decision systems, to make clear what appeals processes might cover a given system’s decision, or to share its mitigation strategy should the system behave in an unexpected and harmful way. The benefits to public agencies of self-assessment go beyond algorithmic accountability: it encourages agencies to better manage their own technical systems, and become leaders in the responsible integration of increasingly complex computational systems in governance.
Goal Four: Strengthen due process by offering the public the opportunity to engage with the AIA process before, during, and after the assessment
The AIA process provides a much needed basis for evaluating and improving agency systems. But without oversight, AIAs could become simply a checkbox for agencies to mark off and forget. That’s why the Algorithmic Impact Assessment process should also provide a path for the public to pursue cases where agencies have failed to comply with the Algorithmic Impact Assessment requirement, or where serious harms are occurring. For example, if an agency fails to disclose systems that reasonably fall within the scope of those making automated decisions, or if it allows vendors to make overboard trade secret claims and thus blocks meaningful system access, the public should have the chance to raise concerns with an agency oversight body, or directly in a court of law if the agency refused to rectify these problems after the public comment period.⁹
Next Steps
As the NYC task force embarks on its study, we hope the Algorithmic Impact Assessment framework can serve as a productive foundation in defining meaningful algorithmic accountability. The task force will be a great opportunity for the public and city agencies to come together to make New York the “fairest big city in America” — that’s why we hope the mayor calls on city agencies to help the task force understand the automated decisions that shape New Yorkers’ lives.
We will be publishing further research on this model in the coming months, and welcome any and all feedback to develop it. And as more jurisdictions take the same first steps New York City has, we hope AIAs will give other communities a useful starting place from which to better understand the systems impacting them, and to design and deploy their own approaches to meaningful algorithmic oversight and accountability.
Thank you to Chris Bavitz, Hannah Bloch-Wehba, Ryan Calo, Danielle Citron, Cassie Deskus, Rachel Goodman, Frank Pasquale, Rashida Richardson, Andrew Selbst, Vincent Southerland, and Michael Veale for their helpful comments on the AIA framework and this post.
[1] Europe has already been developing approaches under various long-standing directives and conventions and the more recent General Data Protection Regulation (GDPR), while the Massachusetts legislature has taken up similar questions, but with a bill focused specifically in the criminal justice context.
[2] See generally, Citron, Danielle Keats. “Technological due process.” Wash. UL Rev. 85 (2007): 1249.; Edwards, Lilian, and Michael Veale. “Slave to the Algorithm? Why a ‘Right to an Explanation’ is Probably Not the Remedy You are Looking for.” 16 Duke L. & Tech. Rev. 18 (2017); Brauneis, Robert, and Ellen P. Goodman. “Algorithmic transparency for the smart city.” (2017); Citron, Danielle Keats, and Frank Pasquale. “The scored society: due process for automated predictions.” Wash. L. Rev. 89 (2014): 1.; Selbst, Andrew D., and Julia Powles. “Meaningful information and the right to explanation.” International Data Privacy Law 7, no. 4 (2017): 233–242.; Diakopoulos, Nicholas. “Algorithmic-Accountability: the investigation of Black Boxes.” Tow Center for Digital Journalism (2014).; Barocas, Solon, and Andrew D. Selbst. “Big data’s disparate impact.” Cal. L. Rev. 104 (2016): 671.; Crawford, Kate, and Jason Schultz. “Big data and due process: Toward a framework to redress predictive privacy harms.” BCL Rev. 55 (2014): 93.
[3] Some have argued that the GDPR’s actual definition, which says that people have the right not to be subject to decisions based on decisions made “solely” by automated processing, introduces a loophole for systems that have any degree of human intervention. Recently released guidelines on GDPR have attempted to adjust for this by requiring that human intervention be “meaningful” rather than a “token gesture,” and requiring that data controllers discuss human involvement in their data protection impact assessments.
[4] https://gdpr-info.eu/art-4-gdpr/
[5] The original draft of Int. 1696 relied on a transparency approach directed towards individuals, allowing individuals to audit decisions made using their own personal information. Though there may be benefit to an individual having that access, it is insufficient to uncovering larger systemic issues. See, for example. Ananny, M., & Crawford, K. (2016). “Seeing without knowing: Limitations of the transparency ideal and its application to algorithmic accountability.” New Media & Society, 1461444816676645.
[6] There might be privacy or security concerns related to making community or group-level data available to researchers. Working out protocols for appropriate disclosures and access controls for researchers will be important for the stakeholders in this process.
[7] In line with our past recommendations, we need a definition of “external researchers” that includes people from beyond computer science and engineering. It should effectively include (at minimum) university researchers from a broad array of disciplines, civil society organizations who can represent the interests of relevant communities, and journalists. This, and other parts of our framework, will be treated in our future work.
[8] See, for example, the Conference on Fairness, Accountability, and Transparency (FAT*)
[9] We see this process as analogous the US’ process for managing the potential environmental impacts made by federal agencies. Under the National Environmental Policy Act, federal agencies must conduct a brief environmental assessment of a proposed action or, if necessary, create a longer environmental impact statement, which the public can challenge before the action takes place. A similar process should take place before an agency deploys a new, high-impact automated decision system. Ideally, agencies would welcome and facilitate this process by identifying stakeholders ahead of time and conducting consultations on critical questions and concerns. Then, after incorporating these concerns in a public notice, any unresolved concerns could be raised during the comment process.