ETHICS, ORGANIZING, AND ACCOUNTABILITY
On October 16, the AI Now Institute at NYU hosted its third annual AI Now Symposium to a packed house at NYU’s Skirball Theatre. The Symposium focused on three core themes: ethics, organizing, and accountability. The first panel examined facial recognition technologies; the second looked at the relationship of AI systems to social inequality and austerity politics; and the final panel ended with a positive look at the intersection of research and labor organizing. You can watch the full event here.
AI Now co-founders Kate Crawford and Meredith Whittaker opened the Symposium with their customary short talk about the the year in AI. It began with a large visualization that sampled just some of the major events that have happened in the last 12 months. Below is an excerpt from that talk.
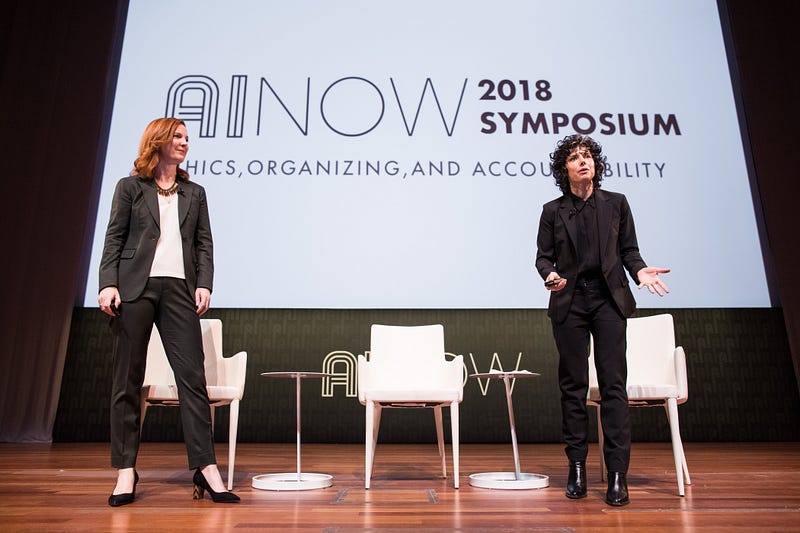
This is our biggest gathering of the year, we review what is happening in AI and its wider implications, recognize good work, and map the paths forward. This year, our focus is on three core themes: ethics, organizing and accountability.
But before we get there, let’s briefly review what has happened this year. AI systems continue to increase in power and reach, against a stark political backdrop. Meanwhile, there have been major shifts and upheavals in the AI research field and the tech industry at large.
To capture this, we worked with Varoon Mathur, AI Now’s Tech Fellow, to visualize some of the biggest stories of the last 12 months. We began by building a database of significant news addressing social implications of AI and the tech industry. The image below shows you just a sample of that — and the result confirmed what many of us have been feeling. It’s been a hell of year.
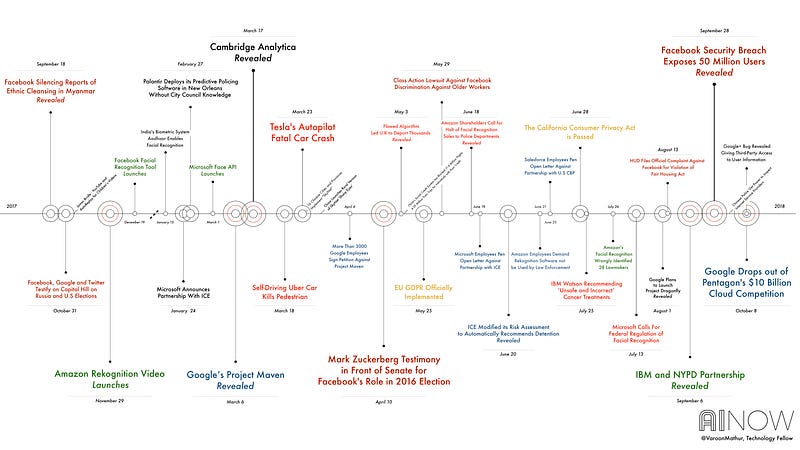
In any normal year, Cambridge Analytica would have been the biggest story. This year, it’s just one of many. Facebook alone had a royal flush of scandals, including a huge data breach in September, becoming the subject of multiple class action lawsuits for discrimination, accusations of inciting ethnic cleansing in Myanmar, potential violations of the Fair Housing Act, and hosting masses of fake Russian accounts. Throughout the year, Facebook executives were frequently summoned to testify, with Mark Zuckerberg himself facing the US Senate in April and the European Parliament in May.
But Facebook wasn’t the only one. News broke in March that Google was building AI systems for the Department of Defense’s drone surveillance program, Project Maven. The news kicked off an unprecedented wave of tech worker organizing and dissent. In June, when the Trump administration introduced the family separation policy that forcibly removed immigrant children from their parents, employees from Amazon, Salesforce, and Microsoft all asked their companies to end contracts with ICE.
Not even a month later, it was revealed that ICE modified its own risk assessment algorithm so that it could only produce one result: the system recommended “detain” for 100% of immigrants in custody.
Meanwhile, the spread of facial recognition tech accelerated. Facebook, and Microsoft joined Amazon in offering facial recognition as a service, offering plug and play models. We also learned that IBM was working with the NYPD, and that they secretly built an “ethnicity detection” feature to search faces based on race, using police camera footage of thousands of people in the streets of New York, taken without their knowledge.
And throughout the year, AI systems continued to be tested on live populations in high stakes domains, with some serious consequences. In March, there were fatalities of drivers and pedestrians from autonomous cars. Then in May, a voice recognition system in the UK designed to detect immigration fraud ended up cancelling thousands of visas and deporting people in error. In July it was reported that IBM Watson was producing ‘unsafe and incorrect’ cancer treatment recommendations.
All these events pushed a growing wave of tech criticism, which focused on the unaccountable nature of these systems. Some companies, including Microsoft and Amazon, even made explicit public calls for the US to regulate technologies like facial recognition. To date, we’ve seen no real movement from Washington.
So that’s just a tiny sample of what has been an extraordinarily dramatic year. Researchers like us who work on the social implications of these systems are all talking about the scale of the challenge we now face: there’s so much to be done. But there have been positive changes too — the public discussion around AI is maturing in some significant ways.
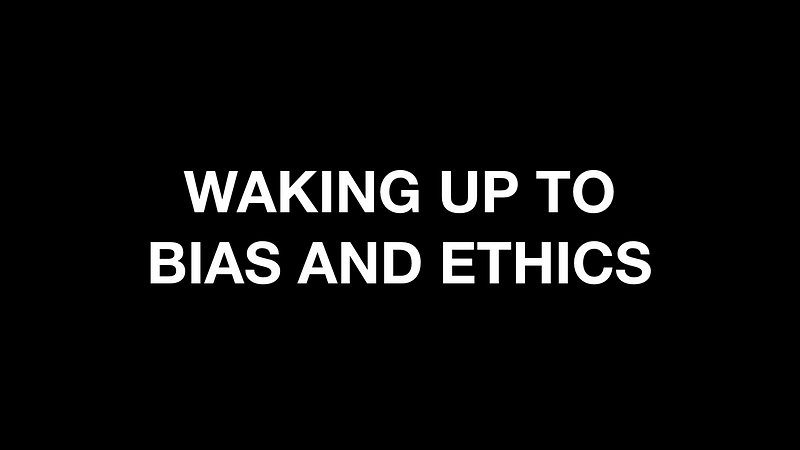
Around six years ago, only a few people like Latanya Sweeney, Cynthia Dwork and a handful of others were publishing articles on bias in AI and large scale data systems. Even three years ago when we held our first AI Now Symposium, bias issues were far from mainstream. But now there is a constant stream of research and news stories about biased results from AI systems. This week it was revealed that Amazon’s machine learning system for resume scanning was recently shown to discriminate against women, even downranking CVs simply for containing the word ‘women.’ And that’s just the latest of many.
Then back in July, ACLU showed how Amazon’s new facial recognition service was incorrectly identifying 28 members of congress as criminals. A significant paper also showed that facial recognition software performs less well on darker skinned women. The co-author of that paper, AI research scientist Timnit Gebru, will be joining us on stage tonight, as will Nicole Ozer, who drove the ACLU project.
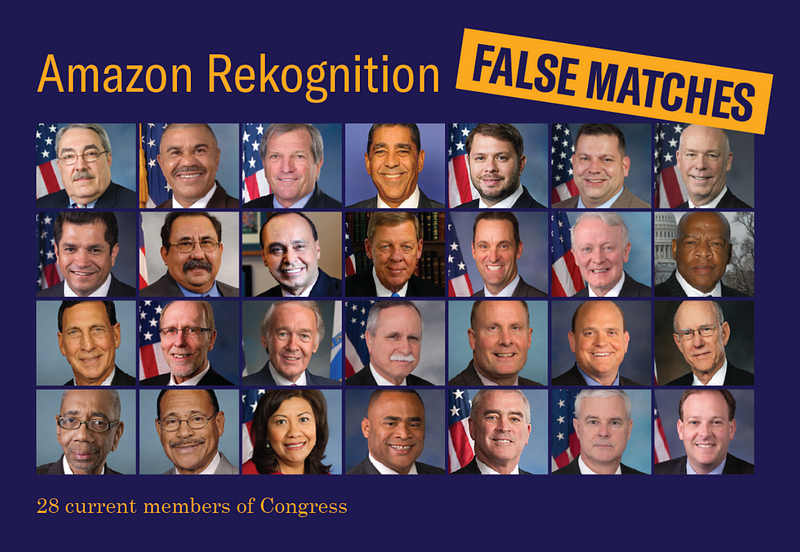
Overall, it’s a big step forward that people now recognize bias as a problem. But the conversation has a long way to go, and it has already bifurcated into different camps. On one side, we see a rush to technical fixes; on the other, we see an attempt to get ethical codes to do the heavy lifting.
IBM, Facebook, Microsoft, and others all released “bias busting” tools earlier this year, promising to help mitigate issues of bias in AI systems using statistical methods to achieve mathematical definitions of fairness. To be clear, none of these tools ‘solve’ bias issues — they are partial and early-stage mitigations. Because at this point, they’re offering technical methods as a cure for social problems — sending in more AI to fix AI. We saw this logic in action when Facebook, in front of the Senate, repeatedly pointed to AI as the cure for problems like viral misinformation.
Technical approaches to these issues are necessary, but they are not sufficient. Moreover, simply making a system like facial recognition more accurate does not address core fairness issues. Some tools, regardless of accuracy, may be unfair to be used at all, particularly if they result in intensified surveillance or discrimination of marginalized groups.
We have also seen a turn to ethics codes across the tech sector. Google published its AI Principles. Microsoft, Salesforce and Axon use ethics boards and review structures. A crop of ethics courses emerged with the goal of helping engineers make ethical decisions.
But a study published recently questioned the effectiveness of these approaches. It showed that software engineers do not commonly change behavior based on exposure to ethics codes. And perhaps this shouldn’t surprise us. To paraphrase Lucy Suchman, a foundational thinker in Human Computer Interaction, while ethics codes are a good start, they lack any real democratic or public accountability. We are delighted that Lucy is joining us on our final panel tonight.
So while ethical principles and anti-bias tech tools can help, much more is needed in order to contend with the structural problems we face.
The biggest, as yet unanswered question is, how do we create sustainable forms of accountability?
This is a major focus of our work at AI Now. We launched officially as an institute at NYU in November 2017, with the goal of researching these kinds of challenges. We have already begun looking at AI in a large-scale context, with an eye to system-wide accountability.
Through this work, 5 key themes have emerged.
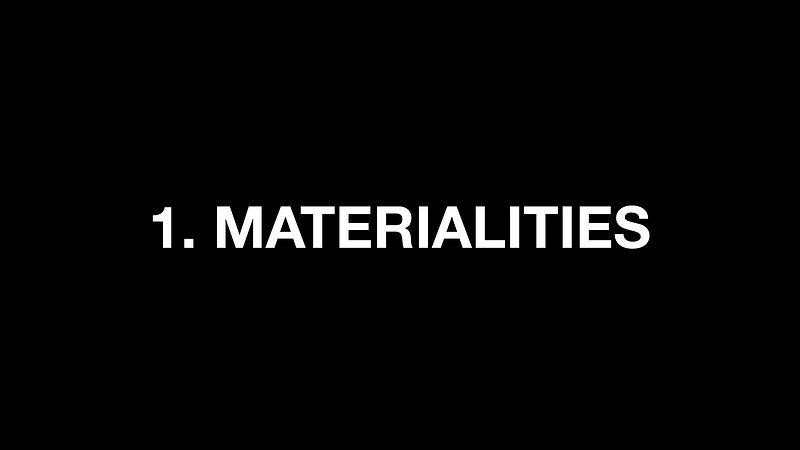
There’s much to learn by examining the underlying material realities of our technical systems. Last month we published a project called The Anatomy of AI. This year-long collaboration between Kate Crawford and Vladan Joler investigated how many resources are required to build a device that responds when you say “Alexa! Turn on the lights.”
Starting with an Amazon Echo, we traced the environmental extraction processes and labor required to build and operate the device. From mining, smelting and logistics, to the vast data resources needed to train responsive AI systems, to the international networks of data centers, all the way through to the final resting place of many of our consumer AI gadgets: buried in giant e-waste rubbish heaps in Ghana, Pakistan and China.
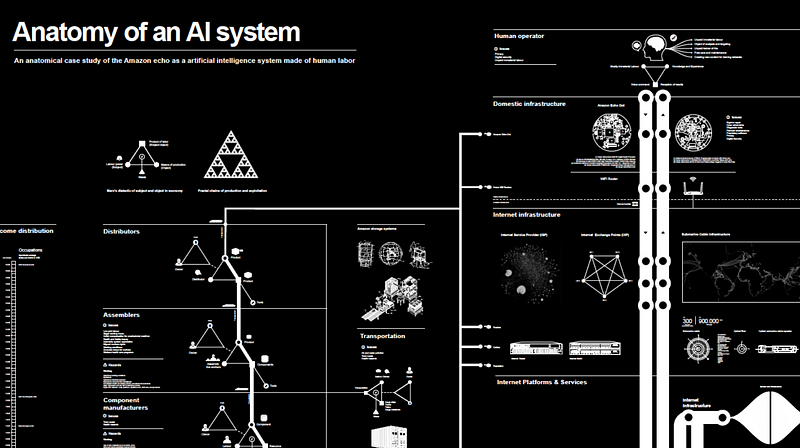
When we look at AI in this way, we begin to see the resource implications of the technologies we use for minor everyday conveniences.
In doing this research, we also discovered there are black boxes stacked on black boxes: not just at the algorithmic level, but also trade secret law and untraceable supply chains. This is part of the reason why the planetary resources needed to build AI at scale are so hard for the public to see.
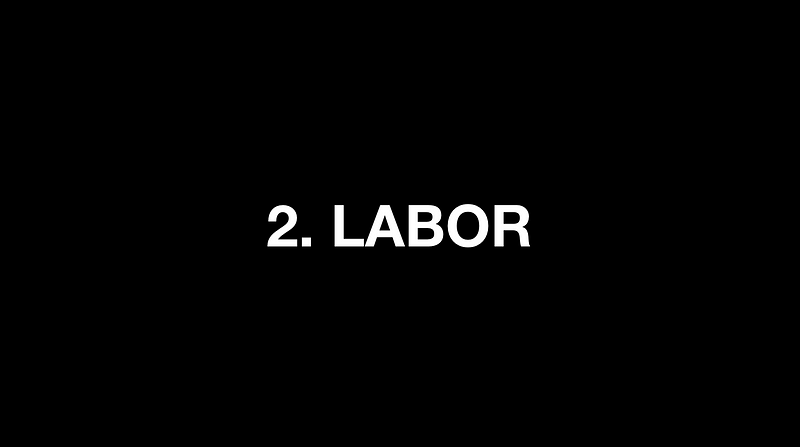
We also continue researching the hidden labor behind AI systems. Often when we think of “the people behind AI” we’re imagining a handful of highly paid engineers in Silicon Valley, who write algorithms and optimize feature weights. But this isn’t the whole picture. As journalist Adrian Chen recently exposed, more people work in the shadow mines of content moderation than are officially employed by Facebook or Google.
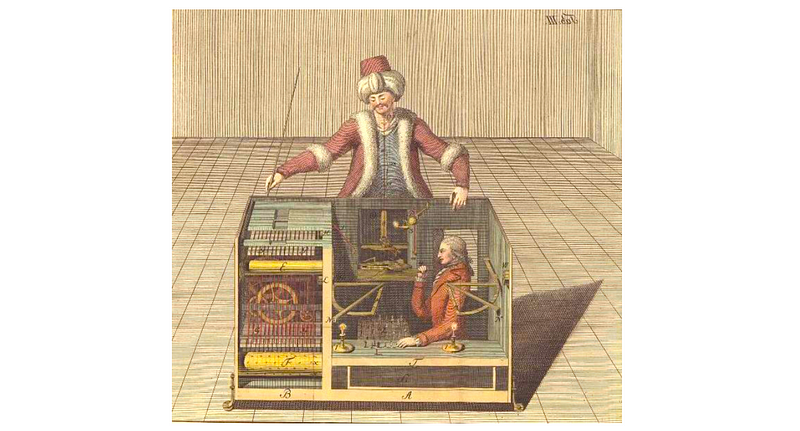
Many scholars are contributing to work in this field, including Lily Irani, Mar Hicks, and Astra Taylor, who coined the term ‘fauxtomation’ to describe those systems that claim to be seamless AI, but can only function with huge amounts of clickworker input. As Taylor observes, the myth of pure automation is about concealing certain kinds of work, and either underpaying for it or pretending it’s not work at all. This is also true of the many AI systems that rely on users to train their systems for free, such as forcing them to click on photos to improve image recognition systems before they can use a service or read a site. Astra will be joining us to discuss this tonight.
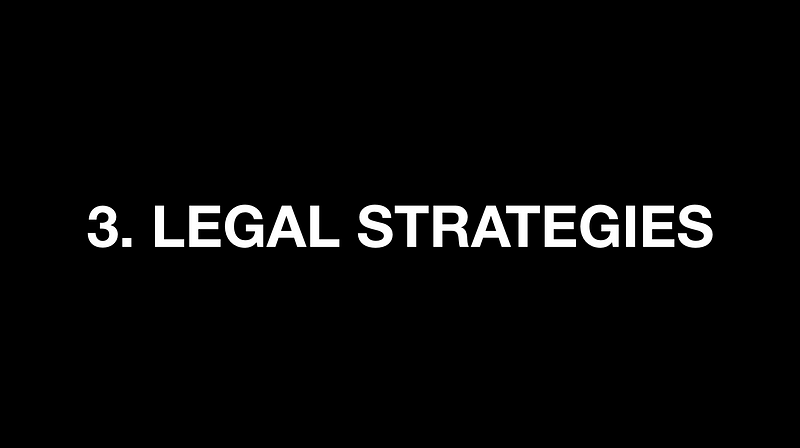
We also need new legal approaches to contend with increased automated decision making. Accountability rarely works without liability at the back end.
We have seen a few breakthroughs this year. The GDPR, Europe’s data protection regulation, went into effect in May. New York City announced its Automated Decision Systems Task Force: the first of its kind in the country. And California just passed the strongest privacy law in the US.
There are also a host of new cases taking algorithms to court. AI Now recently held a workshop called Litigating Algorithms that convened public interest lawyers representing people unfairly cut off from medicaid benefits, who lost jobs due to biased teacher performance assessments, and whose prison sentences were affected by skewed risk assessments. It was an incredibly positive gathering, full of new approaches to building due process and safety nets. Shortly you’ll hear from Kevin De Liban, one of the groundbreaking lawyers doing this work.
We also published our Algorithmic Impact Assessment framework, which gives public sector workers more tools for critically deciding if an algorithmic system is appropriate, and for ensuring more community input and oversight. Rashida Richardson, AI Now’s director of policy research will talk more on this later tonight.
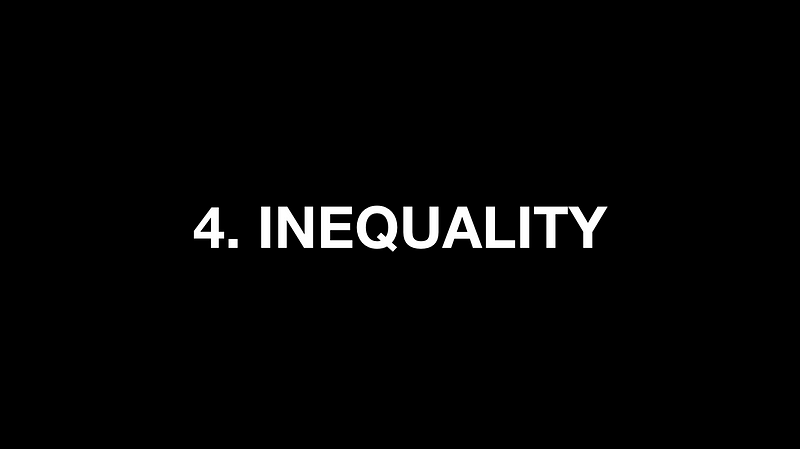
All of this work is engaged with broader systems of power and politics, which raises the topic of inequality.
Popular discussion of AI often focuses on hypothetical use cases and promises of benefit. But AI is not a common resource, available equally to all. There’s growing concern that the power and insights that can be gleaned from AI systems are further skewing the distribution of resources, that these systems are so unevenly distributed that they may be driving even greater forms of wealth inequality. Looking at who builds these systems, who makes the decisions on how they’re used, and who’s left out of those deliberations can help us see beyond the marketing.
These are some of the questions that Virginia Eubanks explores in her book Automating Inequality, and we are delighted that she will also be joining us.
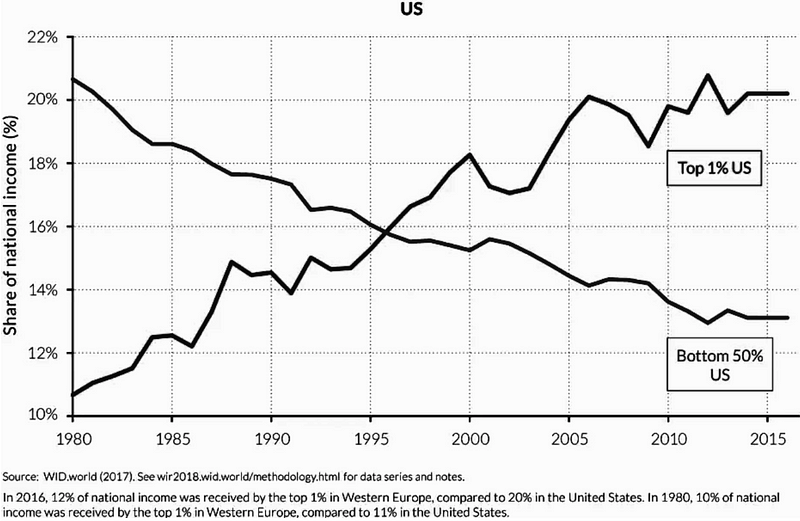
Meanwhile, a new report from the UN said that while AI could be used to address major issues, there is no guarantee it will align with the most pressing needs of humanity. The report also notes that AI systems are increasingly used to “manipulate human emotion and spread misinformation and even hatred” and “run the risk of reinforcing existing biases and forms of exclusion.” We have the UN Special Rapporteur on Extreme Poverty and Human Rights, Philip Alston, joining us to talk about his groundbreaking report on inequality in the US and the role of automated systems.
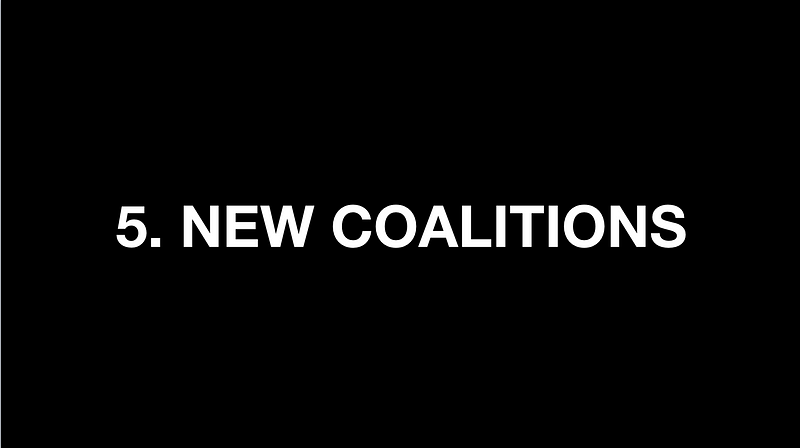
It’s clear that if last year was a big moment for recognizing bias and the limitations of technical systems in social domains, this coming year is a big moment for accountability.
The good news is that work is already happening — people are starting to take action, and new coalitions are growing. The AI field will always include technical research, but we are working to expand its boundaries, emphasizing interdisciplinarity, and foregrounding community participation and the perspectives of those on the ground.
That’s why we are delighted to have speakers like Sherrilyn Ifill, President of the NAACP Legal Defense and Educational Fund, and Vincent Southerland, Executive Director for the Center for Race, Inequality and the Law at NYU, each of whom have made important contributions to these debates.
Genuine accountability will require new coalitions — organizers and civil society leaders working with researchers, to assess AI systems, and to protect the communities who are at most risk.
Because AI isn’t just tech. AI is power, and politics, and culture.