Let’s begin with better regulation, protecting workers, and applying “truth in advertising” rules to AI
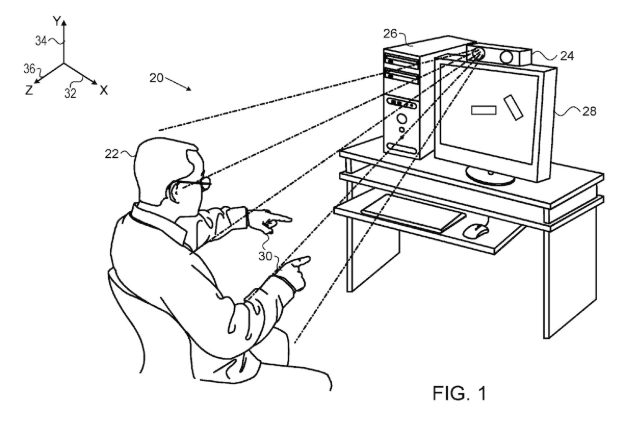
Today the AI Now Institute publishes our third annual report on the state of AI in 2018, including 10 recommendations for governments, researchers, and industry practitioners.
It has been a dramatic year in AI. From Facebook potentially inciting ethnic cleansing in Myanmar, to Cambridge Analytica seeking to manipulate elections, to Google building a secret censored search engine for the Chinese, to anger over Microsoft contracts with ICE, to multiple worker uprisings over conditions in Amazon’s algorithmically managed warehouses — the headlines haven’t stopped. And these are just a few examples among hundreds.
At the core of these cascading AI scandals are questions of accountability: who is responsible when AI systems harm us? How do we understand these harms, and how do we remedy them? Where are the points of intervention, and what additional research and regulation is needed to ensure those interventions are effective? Currently there are few answers to these questions, and existing regulatory frameworks fall well short of what’s needed. As the pervasiveness, complexity, and scale of these systems grow, this lack of meaningful accountability and oversight — including basic safeguards of responsibility, liability, and due process — is an increasingly urgent concern.
Building on our 2016 and 2017 reports, the AI Now 2018 Report contends with this central problem, and provides 10 practical recommendations that can help create accountability frameworks capable of governing these powerful technologies.
Recommendations
1. Governments need to regulate AI by expanding the powers of sector-specific agencies to oversee, audit, and monitor these technologies by domain.
The implementation of AI systems is expanding rapidly, without adequate governance, oversight, or accountability regimes. Domains like health, education, criminal justice, and welfare all have their own histories, regulatory frameworks, and hazards. However, a national AI safety body or general AI standards and certification model will struggle to meet the sectoral expertise requirements needed for nuanced regulation. We need a sector-specific approach that does not prioritize the technology, but focuses on its application within a given domain. Useful examples of sector-specific approaches include the United States Federal Aviation Administration and the National Highway Traffic Safety Administration.
2. Facial recognition and affect recognition need stringent regulation to protect the public interest.
Such regulation should include national laws that require strong oversight, clear limitations, and public transparency. Communities should have the right to reject the application of these technologies in both public and private contexts. Mere public notice of their use is not sufficient, and there should be a high threshold for any consent, given the dangers of oppressive and continual mass surveillance. Affect recognition deserves particular attention. Affect recognition is a subclass of facial recognition that claims to detect things such as personality, inner feelings, mental health, and “worker engagement” based on images or video of faces. These claims are not backed by robust scientific evidence, and are being applied in unethical and irresponsible ways that often recall the pseudosciences of phrenology and physiognomy. Linking affect recognition to hiring, access to insurance, education, and policing creates deeply concerning risks, at both an individual and societal level.
3. The AI industry urgently needs new approaches to governance.
As this report demonstrates, internal governance structures at most technology companies are failing to ensure accountability for AI systems. Government regulation is an important component, but leading companies in the AI industry also need internal accountability structures that go beyond ethics guidelines. This should include rank-and-file employee representation on the board of directors, external ethics advisory boards, and the implementation of independent monitoring and transparency efforts. Third party experts should be able to audit and publish about key systems, and companies need to ensure that their AI infrastructures can be understood from “nose to tail,” including their ultimate application and use.
4. AI companies should waive trade secrecy and other legal claims that stand in the way of accountability in the public sector.
Vendors and developers who create AI and automated decision systems for use in government should agree to waive any trade secrecy or other legal claim that inhibits full auditing and understanding of their software. Corporate secrecy laws are a barrier to due process: they contribute to the “black box effect” rendering systems opaque and unaccountable, making it hard to assess bias, contest decisions, or remedy errors. Anyone procuring these technologies for use in the public sector should demand that vendors waive these claims before entering into any agreements.
5. Technology companies should provide protections for conscientious objectors, employee organizing, and ethical whistleblowers.
Organizing and resistance by technology workers has emerged as a force for accountability and ethical decision making. Technology companies need to protect workers’ ability to organize, whistleblow, and make ethical choices about what projects they work on. This should include clear policies accommodating and protecting conscientious objectors, ensuring workers the right to know what they are working on, and the ability to abstain from such work without retaliation or retribution. Workers raising ethical concerns must also be protected, as should whistleblowing in the public interest.
6. Consumer protection agencies should apply “truth-in-advertising” laws to AI products and services.
The hype around AI is only growing, leading to widening gaps between marketing promises and actual product performance. With these gaps come increasing risks to both individuals and commercial customers, often with grave consequences. Much like other products and services that have the potential to seriously impact or exploit populations, AI vendors should be held to high standards for what they can promise, especially when the scientific evidence to back these promises is inadequate and the longer-term consequences are unknown.
7. Technology companies must go beyond the “pipeline model” and commit to addressing the practices of exclusion and discrimination in their workplaces.
Technology companies and the AI field as a whole have focused on the “pipeline model,” looking to train and hire more diverse employees. While this is important, it overlooks what happens once people are hired into workplaces that exclude, harass, or systemically undervalue people on the basis of gender, race, sexuality, or disability. Companies need to examine the deeper issues in their workplaces, and the relationship between exclusionary cultures and the products they build, which can produce tools that perpetuate bias and discrimination. This change in focus needs to be accompanied by practical action, including a commitment to end pay and opportunity inequity, along with transparency measures about hiring and retention.
8. Fairness, accountability, and transparency in AI require a detailed account of the “full stack supply chain.”
For meaningful accountability, we need to better understand and track the component parts of an AI system and the full supply chain on which it relies: that means accounting for the origins and use of training data, test data, models, application program interfaces (APIs), and other infrastructural components over a product life cycle. We call this accounting for the “full stack supply chain” of AI systems, and it is a necessary condition for a more responsible form of auditing. The full stack supply chain also includes understanding the true environmental and labor costs of AI systems. This incorporates energy use, the use of labor in the developing world for content moderation and training data creation, and the reliance on clickworkers to develop and maintain AI systems.
9. More funding and support are needed for litigation, labor organizing, and community participation on AI accountability issues.
The people most at risk of harm from AI systems are often those least able to contest the outcomes. We need increased support for robust mechanisms of legal redress and civic participation. This includes supporting public advocates who represent those cut off from social services due to algorithmic decision making, civil society organizations and labor organizers that support groups that are at risk of job loss and exploitation, and community-based infrastructures that enable public participation.
10. University AI programs should expand beyond computer science and engineering disciplines.
AI began as an interdisciplinary field, but over the decades has narrowed to become a technical discipline. With the increasing application of AI systems to social domains, it needs to expand its disciplinary orientation. That means centering forms of expertise from the social and humanistic disciplines. AI efforts that genuinely wish to address social implications cannot stay solely within computer science and engineering departments, where faculty and students are not trained to research the social world. Expanding the disciplinary orientation of AI research will ensure deeper attention to social contexts, and more focus on potential hazards when these systems are applied to human populations.
You can read the full report here.