A New AI Lexicon: BIG DATA SWINDLING (大数据杀熟, dà shùjù shā shú)
Jun 29, 2021
Illustration by Somnath Bhatt
One understanding of algorithmic discrimination in China
This is a guest post by Shazeda Ahmed, doctoral candidate at the University of California at Berkeley’s School of Information, and Visiting Scholar at the AI Now Institute.
This essay is part of our ongoing “AI Lexicon” project, a call for contributions to generate alternate narratives, positionalities, and understandings to the better known and widely circulated ways of talking about AI.
Algorithmic discrimination and methods to mitigate it are at the heart of a new, interdisciplinary field of research — one that has had a slow start to questioning whether a universal set of assumptions about what counts as algorithmic discrimination are the same around the world. The Association for Computing Machinery’s Conference on Fairness, Accountability, and Transparency (FAccT), for instance, has only featured a handful of papers investigating how algorithmic fairness critiques vary outside of the Global North. For example, recent FAccT publications have dispensed with “algorithmic fairness” for a more fitting “AI power” framing, and featured projects on ethnographic investigations of data collection practices for predictive policing in Delhi, as well as African expert perspectives on the colonial legacies and pitfalls of sharing data developed across the continent.
In their FAccT paper “Roles for Computing in Social Change,” Rediet Abebe and coauthors advocate for “computing as synecdoche,” meaning that issues at the intersection of information technology and society could draw fresh attention to pre-existing social problems. In Virginia Eubanks’ Automating Inequality, for example, she illustrates that when algorithms have replaced traditional decision-making around child protective services, welfare, and allocating shelter to the unhoused, state institutions can deny people access to vital resources for opaque reasons, propagating what she refers to as the “digital poorhouse.” How does “computing as synecdoche” travel globally? In this essay, I explore “computing as synecdoche” in China, and ask whether this idea has the same power to spark conversations about social inequalities outside of the global North. I look at one example of a phenomenon Chinese observers identify as a form of algorithmic discrimination (“Big Data Swindling”), and explore what changes they want to see as a result.
Foreign observers have long made tacit assumptions that there is no reflection whatsoever on algorithmic discrimination as it affects artificial intelligence (AI) technologies in China. On the contrary, the literal translation from English to Mandarin of terms like black box algorithms (黑箱算法, hēixiāng suànfǎ), or algorithmic discrimination / algorithmic bias (算法歧, suànfǎ qíshì), have increasingly surfaced in Chinese academic, technology industry, policy, and news media sources. Delving deeper into this growing body of work, I encountered culturally situated neologisms that have emerged in China to describe related issues.
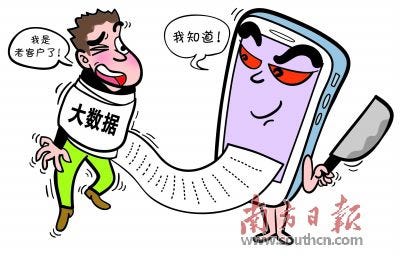
The roughly translated “big data swindling” (大数据杀熟, dà shùjù shā shú), for instance, is a hotly debated term used to describe a mix of dark patterns and dynamic pricing that online platforms employ to exploit users’ differential willingness to pay for goods and services. Suppose two different people use the same ride-hailing app to reserve cabs under roughly similar conditions (factoring in distance, surge pricing, etc.), but one person is assigned a higher fare presumably because the company running the app knows exactly how much more that person is willing to pay based on data recordings of their past purchasing behavior. This “big data swindling” has served as a rallying point around which to criticize Chinese tech platforms’ unchecked dominance.
“Big data swindling” directly translates to “big data-enabled killing through familiarity,” where the latter portion of the phrase (杀熟, shā shú) is an older colloquialism used to describe the act of taking advantage of a close or familiar contact in order to benefit oneself. The phrase tends to describe interactions with relatives and friends, where for example, one might pressure social contacts to ask about vacancies at their jobs when one is seeking employment. When used in conjunction with big data, however, the “familiarity” now comes from massive datasets, which companies have culled from customers’ online activity and use to understand individuals’ behaviors, leading them to pay more for goods and services. Third parties play a crucial role in this process: they mine data on payment history, duration of time spent on a web page, and similar data used for price discrimination. These methods through which an invisible set of actors becomes “familiar” with users in order to exploit that intimate knowledge are by now well-known to people who have followed similar controversies in the United States and Canada.
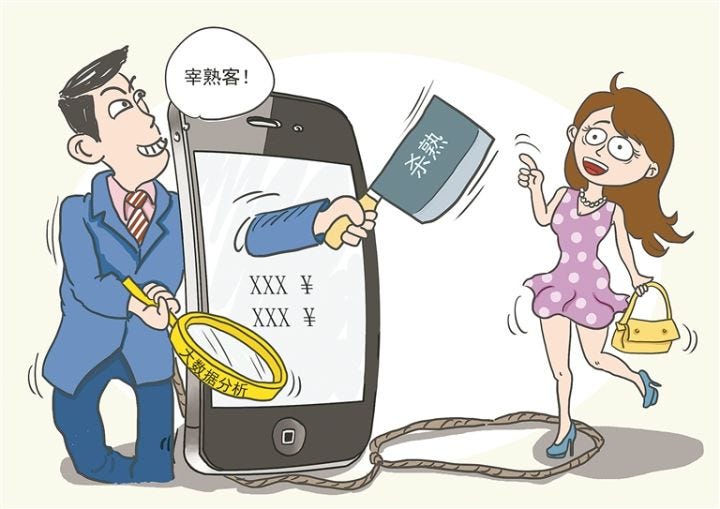
This characterization of “big data swindling” in China is unique for at least two reasons. First, people discovered that it was happening within some of the country’s biggest platforms, such as Didi Chuxing (ride-hailing) and CTrip (travel booking), among others. Around 2018, Chinese social media users on Weibo and Zhihu (a question-and-answer forum similar to Quora) began to notice, document through screenshots, and share examples of how they received different prices in major travel, ride-hailing, e-commerce, and entertainment apps depending on their device, location, and whether or not they were a new user.
Collective efforts to develop what Motahhare Eslami and others have coined “folk theories of algorithms” generated enough public interest in “big data swindling” that in 2019 the Beijing Consumer Association conducted a three-part survey to understand the practice. While 57 percent of respondents claim to have experienced “big data swindling” firsthand across 14 major apps, the study also highlighted that the concealed nature of how users came to see different prices for the same products and services would make it next to impossible to defend users’ rights in court. Companies fired back that “big data swindling” did not exist, and that the discrepancies users highlighted simply reflected new user discounts and real-time price fluctuations reflecting changes in inventory. Similarly, some academics claimed consumers’ self-reported instances of “big data swindling” were unreliable.
Yet enough researchers acknowledge “big data swindling” as a significant problem that dozens of law and economics papers have been published on the topic, spanning experiments to document its existence to recommendations for how Chinese anti-monopoly, e-commerce, and consumer protection legislation can be used to combat it. This abundance of commentary has led Chinese academic literature and media to cite “big data swindling” as an example of algorithmic discrimination that takes place in China, more so than other domestic examples that reflect social inequalities related to class, ethnicity, gender, or related attributes.
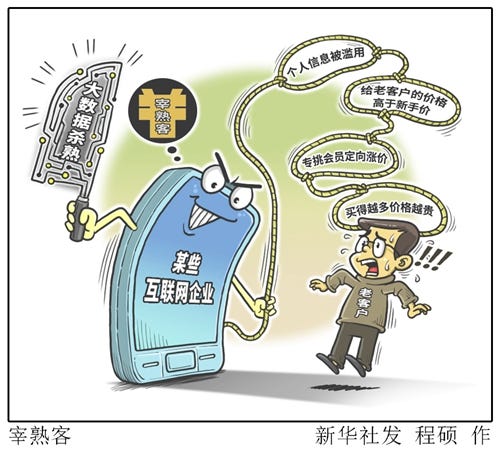
While a growing number of Chinese tech researchers and experts acknowledge algorithmic discrimination as a global problem, most shy away from applying this critique to Chinese tech companies and instead reach for familiar stories in the United States, such as the that of the racially biased COMPAS recidivism risk calculator. Similarly, proposed solutions tend to either fall on the state to regulate “big data swindling” out of existence using antitrust protections, on the companies to ‘self-regulate’ (自律 zì lǜ), or perhaps least realistically, on consumers to “reduce the leakage of [their] personal information onto platforms, delete prior browsing and purchase records in a timely manner, and regularly shop around to compare prices when buying on platforms.” Like much of the abstract language that runs through dominant Western discourse on AI ethics or fairness, there is a similar silence here in acknowledging the inequalities Chinese algorithmic decision-making systems might inflict beyond price discrimination.
The collective uproar over “big data swindling” skirts the underlying socioeconomic issues that make the practice unfair. If location data or specific devices (e.g., using an iPhone) affect pricing, what inequalities are these characteristics proxies for and who, therefore, do they disadvantage? While the discourse about “big data swindling” highlights underlying social issues in the vein of “computing as synecdoche,” it has yet to cross a threshold into open acknowledgement of how price discrimination and dark patterns in the country’s biggest digital platforms could disadvantage China’s poorest and least tech-savvy citizens. Despite the potential “big data swindling” has to shore up broader social critiques or even pressure tech firms to change their practices, it appears to be a less active debate than issues such as growing public awareness of the harsh conditions of food delivery drivers’ algorithmically-managed labor. Yet as these examples of technologically-mediated inequality continue to enter Chinese public discourse, opportunities for locally situated forms of “techlash” may well drive new instances of “computing as synecdoche.”
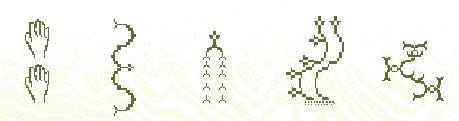
[1] Original Source: “内部员工自曝’大数据杀熟’真相” [“Internal Employees Air the Truth About ‘Big Data Swindling’”], Southern Daily, illustration by 杨佳 [Yang Jia], April 19, 2019. http://it.people.com.cn/n1/2019/0419/c1009-31038238.html
[2] Original source: “对电商平台’大数据杀熟’就应立法禁止” [“Legislation Should Ban E-commerce Platforms’ ‘Big Data Swindling’”], posted to Zhihuby user 江德斌just [Jiang Dewen just], July 5, 2018. https://zhuanlan.zhihu.com/p/39111439.
[3] Original source: “新华时评:管住大数据杀熟的手” [“Xinhua Commentary: Controlling the Hand of Big Data Swindling”], Xinhua, illustration “宰熟客” [“Cheat Familiar Customers”] by 程硕 [Cheng Shuo], April 8, 2019. http://www.xinhuanet.com/fortune/2019-04/08/c_1210102776.htm